For manufacturers navigating complexities of artificial intelligence (AI), one key challenge is balancing the advantages of new technology with immediate and long-term costs.
Reducing Manufacturing Cost and Risk with Hybrid AI
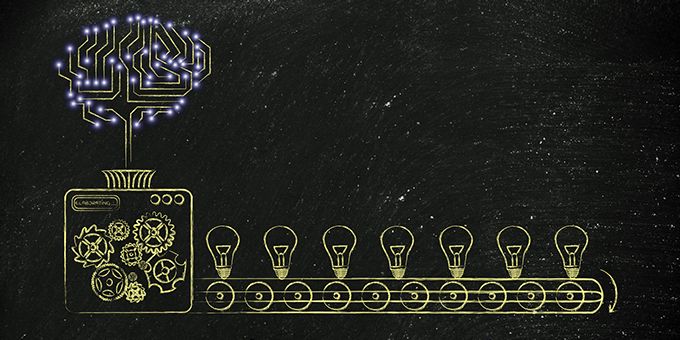
Jonathan Hou | Pleora Technologies
While some approaches force a propriety “locked-in” solution, and others require a complete infrastructure overhaul, hybrid AI allows a gradual migrate to more advanced AI inspection without disrupting existing processes.
Understanding AI and Its Challenges
Before getting too far, we should understand the different terminology related to AI. Broadly, AI is a machine solving a problem in a way that we consider intelligent. Machine learning is a branch of AI where computers are provided with a set of data and learn how to solve a specific problem without human intervention.
Deep learning then expands machine learning methods to powerful neural networks that are inspired by the way our brains function. With training and labelling, a user teaches the system how to make a decision based on good or bad outcomes (for example, pass/fail). The neural network can then starting analyzing the data and making its own decision.
Image 1: The branches of AI lead to machine-based decision making.
While AI has the promise of improving inspection results, manufacturers evaluating the technology consistently highlight a few key factors that potentially limit their ability to deploy solutions.
Lack of Technology Infrastructure to Support AI
This is a key concern, in particular for manufacturers with a significant investment in inspection equipment. A major automotive manufacturer, for example, has over 2000 cameras completing 2 million inspection tasks per day on just one production line. Imagine the costs of replacing cameras, vision controllers and deploying new applications to support AI.
Training Complexity and Costs
End-users often cite concerns related to potential costs of contracting or hiring expertise to develop AI algorithms. New “no code” algorithm development platforms and off-the-shelf plug-ins for common inspection tasks significantly streamlines design, while edge processing hardware can provide data for continuous model training.
Frontline Processes
Manufacturers often have decades of human and technology based processes that deliver proven results. Re-training humans on new software and interfaces is costly and time-consuming, while replacing existing processes is risky.
Why is AI important for Brands?
There are a few key reasons why brand owners and manufacturers are investigating AI:
-
Increase yield and profit;
-
Inspect materials not handled well by traditional computer vision, such as fabric, metal, and glass;
-
Poka-yoke, the Japanese term for mistake proofing, uses automation to make an error obvious to a human. Digitizing manual work instructions, for example, can highlight if a product has been properly packaged before it’s shipped to the consumer. A recent 2021 study highlighted a 62% potential error rate when humans follow manual assembly instructions.
Hybrid AI is particularly well-suited for a few key markets. The consumer goods market is already heavily invested in machine vision quality inspection. Layering AI capabilities on top of existing computer vision processes reduces false-positives, where even a few percentage points of improvement significantly reduces product waste, lowers costs related to secondary screening, and increases production uptime.
For metal fabrication, such as automotive parts, AI improves error proofing, dimensional verification, and surface quality processes that rely on a combination of automated and human inspection. Humans are well-suited for inspection tasks, but when we get tired or distracted AI is a powerful tool to help alert us on issues and guide decision-making.
AI is being used in the food and beverage market to reduce errors, ensure product safety and avoid recalls, and automate manual tasks. In sorting, brand evaluation, and packing applications, AI can automate or add decision-support for human processes. With new sensor technologies, such as hyperspectral imaging, manufacturers can add advanced inspection for grading and product characterization, verifying product mix, and screening for defects and foreign materials.
In the print and packaging industry, AI is increasing automation – from detecting printing and labelling errors to counting and sorting – as well as training custom “short run” packaging inspection with different threshold requirements without requiring extensive configuration or line down time.
Image 2: There are many different reasons to use AI, from improving yield to automating manual inspection tasks. For packaging, AI is well-suited for detecting labelling errors.
What is Hybrid AI?
Hybrid AI merges the best of traditional rules-based computer vision with advanced AI inspection capabilities to help improve accuracy in existing systems, without adding deployment complexities and infrastructure costs.
Classical Computer Vision vs. AI
In a classic computer vision application, a developer manually tunes an algorithm for a task to be completed. This can require significant customization if products A and B have different thresholds on what is considered an error. Inaccuracies may generate excessive false positives that stop production and force costly manual secondary inspection, or missed errors that result in defective or poor quality products going to market. The advantage with classical algorithms is that you don’t require data to get started.
Similarly, AI algorithm training has traditionally required multiple time-consuming steps and dedicated coding to input images, label defects, fine-tune detection, and optimize models. More recently, no-code software platforms provide an intuitive drag-and-drop approach to develop AI applications. More advanced platforms provide full flexibility for developers to code and test algorithms, mix computer vision and AI, or even load the latest open source models.
Hybrid AI Deployment Strategy
Leveraging embedding processing and a hybrid architecture, existing machine vision inspection systems infrastructure can upgraded with AI. In this deployment strategy, the embedded device acts as an intermediate device between the camera and host PC.
The embedded device “mimics” the camera for existing applications and automatically acquires the images and applies the AI algorithm on top of the camera feed. Processed data is sent over GigE Vision to the inspection application, which receives it as if it were still connected directly to the camera. This allows the re-use of existing cameras, controllers and end-user applications. The embedded device can be programmed to save incoming images for continuous offline training to improve inspection results.
Image 3: Hybrid AI simplifies training and deployment, while allowing reuse of existing infrastructure and processes.
Total Cost of Ownership
Cost is a driving factor in any new technology implementation, with hybrid AI delivering a few significant advantages over deploying a new AI-based inspection system. When evaluating the two approaches, it’s important to consider re-use of existing hardware and applications, lower training and retraining costs for algorithms and processes, the ability to use open source and third-party AI frameworks, and perpetual licensing. Depending on variables, total cost of ownership for a new system can be five times more expensive than a hybrid approach for most manufacturers.
What’s Next?
AI is an important part of an overall Industry 4.0 initiative for manufacturers seeking ways to leverage data to streamline supply chains. Specific to quality inspection, forward-thinking businesses are looking at the cloud as a way to share algorithms across global sites to ensure consistent product quality and analytics-based decisions.
Hybrid AI lets brand owners quickly realize the benefits of AI for quality inspection and automation, without disrupting investments in infrastructure and processes, while creating a stepping stone towards more comprehensive process monitoring and Industrial Internet of Things (IIoT) integration.
The content & opinions in this article are the author’s and do not necessarily represent the views of ManufacturingTomorrow
Comments (0)
This post does not have any comments. Be the first to leave a comment below.
Featured Product
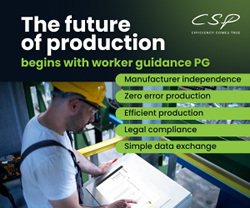