New data- and ML-based business models will become more commonplace, so early adopters will have a competitive advantage. Machine learning will happen both in the cloud and on the edge, i.e., directly on or next to production machines and sensors.
The Impact of AI and Machine Learning Technologies in Manufacturing
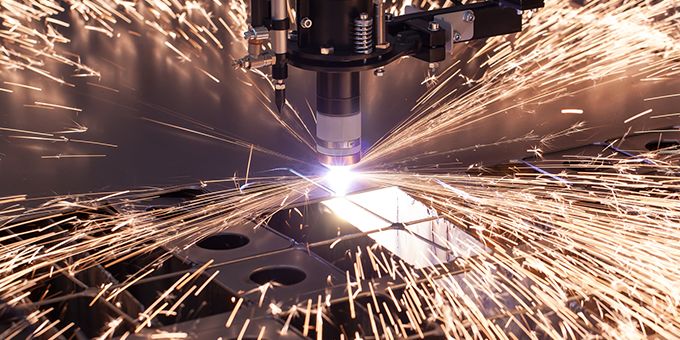
Q&A with Ralf Klinkenberg, Co-Founder and General Manager | RapidMiner
Tell us about yourself and RapidMiner.
My name is Ralf Klinkenberg. I am a data-driven entrepreneur with more than 30 years of experience in machine learning and advanced data analytics across various industries.
In 2007, Dr. Ingo Mierswa and I founded RapidMiner, which grew from 2 founders in 2007 to 100 employees today. Our user community has grown to more than 770,000 registered users in over 150 countries and more than 1,000 universities worldwide use our platform for education and research.
RapidMiner is an end-to-end machine learning platform. It’s designed to help users conduct advanced data analysis from the ground up, supporting everything from gathering and prepping relevant data to training models that provide insights and predictions about the shop floor. Production teams can use our platform to ensure they have the right data, automate model creation, and test the efficacy of their models before deploying them into production.
What are some of new Artificial Intelligence and Machine Learning technologies that you think will have a big impact in manufacturing this year?
There are a few technologies that we see as up-and-coming in the manufacturing realm:
- Automated and Semi-Automated Data Cleaning. Guided analytics to support data cleaning and preparation, which prevent inaccurate data from impacting models and ensure accurate forecasts.
-
Automated and Semi-Automated Feature Engineering and Feature Marts. Feature engineering uses domain expertise to pull relevant inputs from raw data. It provides relevant context, makes models more understandable, and increases their accuracy.
-
Explainable ML Models and Explainable Classifications and Predictions. Models are only trusted and deployed if they can be understood so that you can be sure you’re adding value, not risk.
-
Specialized Auto Modeling Tools. Automated modeling further eases and accelerates the modeling process by helping teams select and create the right models for predictive maintenance, yield optimization, and other common use-cases.
Overall, we will see more machine learning being used in the ML tools themselves, to automate them more, to support the user more, and to further accelerate model generation, deployment, and operation. I also expect to see a further industrialization of data science: produce and deploy more models faster with less time and effort needed per model, and more automation and value creation.
Right now, we’re in a place where new technologies are getting adopted in a piecemeal fashion, to do very specific jobs. Do you think at some point we’ll see a paradigm shift to an AI/ML-first mindset?
Yes, eventually we will see a shift to a more data-driven and machine-learning-oriented mindset. For example, when designing products and production processes, engineers will consider which data to collect and for which purposes ahead of time.
This will help to continuously improve and optimize products and production processes. Data will be captured before the design of a product (automated and semi-automated market research), during all stages of its design and production, across the whole supply chain, and for the whole product lifetime. This facilitates the detection of market needs and demands earlier, allowing manufacturers to optimize products, increase product quality and market fit, and improve efficiency and customer satisfaction as a result.
New data- and ML-based business models will become more commonplace, so early adopters will have a competitive advantage. Machine learning will happen both in the cloud and on the edge, i.e., directly on or next to production machines and sensors.
Solutions that are narrowly focused on only one use case will become less relevant. On the other hand, flexible, enterprise-grade industrial data science platforms will become more widely used, integrating data from many diverse sources and supporting many use cases within the same framework and graphical user interface.
What are some of the problems and challenges you see on the horizon to adopting this technology?
Current problems are a high entry barrier (not everyone is a data scientist) and a lack of transparency and understandability. Data science needs to be understandable and produce models and predictions that are both trustworthy and explainable.
At RapidMiner, we’ve worked hard to enable more and more user types (not only data scientists and programmers, but also domain experts like process engineers, business analysts, managers, etc.) to optimally support cross-functional teams so that they can collaborate, share knowledge, and support each other on machine learning projects.
We want to enable everyone to positively shape the future with AI. In order to lower the entry barrier, we provide a variety of tools: automated model creation and optimization, reusable application templates, built-in recommendations for the next best process design step, and other guided analytics tools.
Additionally, programmers can seamlessly integrate their Python or R code into RapidMiner processes so that non-coders can benefit from their work. Coding is optional, but seamless code integration into visual workflows further facilitates collaboration between heterogeneous teams inside the platform.
In our research team, we also work on further industry-specific guided analytics tools, tailored to the needs of the manufacturing industry, to ease and accelerate the process from idea to deployment for many different use cases.
In order to ensure that models and their predictions are explainable, we offer algorithms that generate understandable models and explanations. There’s also a simulator that allows users to experiment with various what-if scenarios for better model understanding, and an optimizer that finds the best process parameters and model inputs to optimize for your desired outcome (less defective products, avoiding unexpected downtime, lowest production cost, etc.).
Share with us some of the challenges that are unique to manufactures when they try to implement AI/ML solutions?
The complexity of the manufacturing domain, the variety of data sources and relevant aspects, the high number of potential influence factors, and often very complex correlations over various time horizons make most manufacturing use cases hard to solve. For example, standard customer analytics use cases like direct marketing optimization or customer churn prediction require fewer data sources and most often have simple correlations.
There rarely is a one-size-fits-all solution. Every manufacturing company is different. Every shop floor and machine park and set of products differs. Most of the time a lot of domain expertise is needed to solve these tasks, e.g., to consider the relevant data sources and generate meaningful features. Because of this complexity, a one-size-fits-all automated approach is usually not sufficient without a detailed understanding of the source data, the production process, and the produced products (i.e., what’s happening on the shop floor).
In our experience, a combination of manufacturing expertise and data science expertise leads to the best results. Thus, enabling manufacturing engineers and domain experts to perform data science with easy-to-use tools and guided analytics is a powerful approach.
The effort is not to replace manufacturing experts but to enable them to leverage machine learning to efficiently make the right decisions at a large scale. This is why we focus on helping customers upskill their staff—we want to enable data-loving people of all skill levels to leverage the value-creation opportunities machine learning has to offer.
Do you have any promising use cases that you’re seeing in manufacturing today, and do you see that shifting in the future?
Yes, we see a large variety of machine learning use cases in manufacturing and the number is constantly growing. Here are some examples:
-
Demand forecasting. How much of which of my products will be ordered when? How much do I need to order from my suppliers and when? How much energy will I need for the production and when? Etc.
-
Predictive maintenance. Predicting machine failures before they happen to prevent them, reducing failure risks and maintenance costs, while also improving process reliability. Predictive maintenance can be used for your manufacturing machines as well as in your own products during their product lifetime.
-
Product quality prediction and optimization. Reducing costs for base products, increasing resource efficiency and energy efficiency, reducing overall costs, increasing process reliability, increasing customer satisfaction, and profitability.
-
Mixture of ingredients optimization. What is the best mixture of ingredients to achieve the desired product properties and quality at the lowest possible cost in a reliable way?
-
Supply chain risk prediction and supply chain optimization. Increase resilience, lower costs, react faster to unusual events (e.g., by monitoring online media).
In the future, we will also see more complex use cases and applications. A few examples include:
-
Instead of single use cases based on a single production step and data source, we will see more data integration along the whole production process, departments, and companies. Therefore, quality issues occurring later in the production process or in the field can be traced back to the problem source—no matter how many steps earlier in the process it was and possibly even when the source is at one of the suppliers.
-
Collaboration and optimization across entire supply chains and value creation networks will also become common. We are currently working on joint research projects with various companies from the automotive and manufacturing industry to create and validate a platform for this purpose.
-
An industrialization of data science with pre-build easily configurable analysis modules for various use cases and more guided analytics for non-data scientists.
How has the current pandemic affected manufacturers efforts to implement AI/ML, and do you think those trends will last beyond the pandemic?
In some cases, the pandemic may have slowed down some AI & ML projects. However, in most companies, we now see increased efforts to quickly digitize the shop floor – everything from the manufacturing process and product lifecycle to supply chain and network. This is all in an effort to increase robustness and resilience (to be better prepared for the next pandemic or supply chain break-down), improve efficiency and product quality, and increase profitability.
A further digitization of the production process, more sensors, and better data analysis increase the speed of detecting issues, provide more insight into root causes, and reduce reaction times. Additionally, they not only reduce incidence costs but also increase the agility and success of the companies investing in these areas. A lot of companies now systematically select the most promising use cases and implement them—not just one use case, but often several in parallel.
Overall, the adoption speed of AI and ML has increased significantly. And we expect this trend to continue after the pandemic, as companies aim to become more data-driven, faster. Whoever stays behind will risk losing to the competition and becoming obsolete faster than they might expect.
About Ralf Klinkenberg
Ralf Klinkenberg, co-founder and head of research at RapidMiner, is a data-driven entrepreneur with more than 30 years of experience in machine learning and advanced data analytics research, software development, consulting, and applications in the automotive, aviation, chemical, finance, healthcare, insurance, internet, manufacturing, pharmaceutical, retail, software, and telecom industries. He holds Master of Science degrees in computer science with focus on artificial intelligence, machine learning, and predictive analytics from Technical University of Dortmund, Germany, and Missouri University of Science and Technology (MST), Rolla, MO, USA. In 2001 he initiated the open-source data mining software project RapidMiner and in 2007 he founded the predictive analytics software company RapidMiner with Dr. Ingo Mierswa.
The content & opinions in this article are the author’s and do not necessarily represent the views of ManufacturingTomorrow
Comments (0)
This post does not have any comments. Be the first to leave a comment below.
Featured Product
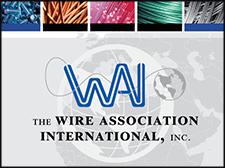