The secret lives of manufacturing defects - How defects pass through the system undetected
2023 saw a number of high-profile recalls linked to manufacturing defects. Despite technological improvements in the field of quality inspection, many safety-critical industries witnessed an increase in the number of defects and recalls. Here Zohar Kantor, chief revenue officer at quality inspection pioneer QualiSense, traces how defects enter the manufacturing process and avoid detection.
It is now over a decade since the largest recall in the history of the automotive industry. In 2013, several automakers began significant recalls due to defect Takata airbags. In 2017, the Japanese parts manufacturer was forced to file for bankruptcy when the amount of money due in compensation was more than was required for its survival.
Despite the costs of recalls and the strenuous efforts of quality managers and other production professionals, manufacturing defects are still wreaking havoc a decade on. In May 2023, for example, more than 30 million cars were included in an airbag recall issued by the US National Highway Traffic Safety Administration.
Given the costs of defects and the efforts made by manufacturers to detect them, how are so many defects able to avoid detection?
The limits of manual inspection
Defects can begin at any stage of the manufacturing process due to a wide range of factors. It is generally unusual for a defect to be detected at source, instead defective parts are only spotted at the end of the production line where in many cases, they are extremely challenging to spot.
Despite modern developments in machine vision and AI, many manufacturers still rely on human inspectors. Manual inspectors have to work at incredible speed and under continuous pressure. The human eye is remarkable, one of the most complex and sophisticated organs evolved however Human beings are not immune from tiredness, lack of concentration or cognitive bias and a proportion of defective parts inevitably escapes capture at this critical stage. Estimates vary, but most research places the mistake rate anywhere between ten to twenty per cent when using manual inspectors.
Depending on the industry, different inspection methods are adopted. If you are only using sampling, rather than inspecting every part individually, it is easy to see how a rarer defect would evade capture. In safety-critical industries though, every part will be inspected and given the shortcomings of manual inspection, many companies have invested in technology to improve the odds of detection.
Investing in AI
AI based quality inspection systems are vastly superior in detecting defects, but most of the existing technology in this area has its limitations. Firstly, the AI system relies on hardware like imaging and other sensors. Relatively subtle changes in environmental conditions, like lighting for example, can create problems here.
The more basic algorithms are only effective at catching simple defects. Whether an AI can spot a defective part before it leaves the plant depends on the sophistication of the algorithm and the use case. Basic rule-based algorithms will only suffice for inspection challenges that are deterministic and the environment is repetitive in nature, but most modern production processes, particularly in safety-critical industries like aerospace, automotive and med-tech, require deep learning algorithms.
For training these AI models, the process is often lengthy and time-consuming. The end result might be a model that is good at detecting common defects, as the model will have seen lots of examples of these. However, we run up against the problem referred to by AI technologists a ‘‘class imbalance,’’ where common defects routinely show up in the training, but rare defects do not. Not surprisingly, the end result is a model therefore fails to catch the outliers.
Continuing to avoid capture
Due to errors routinely made by manual inspectors and the shortcomings of existing AI, components with minor defects leave the plant. End of line detection is generally effective for single components, but when you go to assembly you have another layer. When multiple components are assembled together, defects that went undetected at an earlier stage of production can often continue to avoid detection. Imagine a simple single gear. A minor defect is not spotted on the surface, as sheet inspection of metal surfaces is notoriously challenging. This gear then becomes part of a transmission, which in turn becomes part of a car.
Functional testing of the product may expose a defect in some instances, but in many cases the defective component, buried inside the assembled product, will continue to escape attention at this stage. The defect can now pass through multiple stages of the production process undetected, as it is no longer visible even with the most thorough inspection process and the most sophisticated AI.
The point at which the defect comes to the attention of the manufacturer is when the product fails within its warranty period or worst still, is shown to violate regulatory requirements. The longer the defects evades detection, the bigger the cost to the manufacturer, as you now have to recall the entire product rather than simply addressing the defective part in isolation.
The next step in AI
As already observed, although AI has assisted in improving defect detection, existing systems still have their limitations. Thankfully, that is now beginning to change. The hardware that inspection systems rely on is both improving and becoming affordable to more companies. At the same time, we are overcoming the challenges of training AI models for inspection, by automating the process of model training which makes the process faster and more accurate. It is the quality manager that transfers his knowledge in the model creation process, and with augmented AI, the quality manger can easily continue to update the model on the production line, therefore preparing for the most challenging inspection tasks.
To find out more about AI and visual inspection, visit qualisense.ai
Comments (0)
This post does not have any comments. Be the first to leave a comment below.
Featured Product
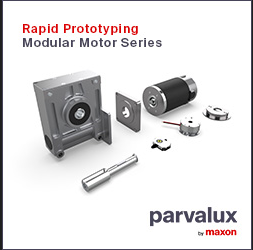