Without any external input to guide its actions or decisions, a robot would behave like a machine, performing tasks without adaptation or autonomy. Serving as the critical link between the robot and its environment, sensors enable the robot to perceive and interpret.
The Core of Optimizing Robotic Performance: Sensors in Warehousing
Heico Sandee, Founder & CEO | Smart Robotics
A crucial factor that distinguishes a robot from a machine is its ability to respond to external input or stimuli. Without any external input to guide its actions or decisions, a robot would behave like a machine, performing tasks without adaptation or autonomy. Serving as the critical link between the robot and its environment, sensors enable the robot to perceive and interpret.
Applications across a spectrum
The importance and purpose of sensors, however, vary greatly between different types of robots. Whereas the traditional manufacturing robot arms, for example, make little use of sensors and typically just follow a pre-programmed path, on the other side of the spectrum there are mobile robots that work in predominantly human environments (e.g. robot carts that drive around hospitals delivering laundry) relying heavily on sensors. It’s possible for the traditional approach to work the way it does because everything around the robot is fixed — enclosed within a cell, human access is restricted, and the items handled by the robot remain consistently positioned in the same location. In contrast, its sensor-heavy counterpart operates in dynamically changing environments, where unexpected human presence can suddenly obstruct the robot’s path. To operate in environments such as these, 3D cameras, lidars, sonars and so on equip robots to build an understanding of their environment and update it constantly.
The requirements for a robotic solution for a logistic warehouse falls somewhere in the middle— the environment doesn’t change all that much, but the items that need to be handled change with each task and their positions too, are often uncertain. Utilizing 2D and 3D cameras, in this case, proves highly advantageous, especially to find these items. Let’s look at a few applications of such technologies that improve robotic reliability and efficiency in a warehouse setting.
Understanding the surroundings
3D cameras can be used in pick and place systems to capture detailed images that help the robot develop a comprehensive understanding of its surroundings. Vision algorithms then process this data, enabling the robot to perform its pick and place tasks with accuracy and efficiency. By continuously monitoring and evaluating its surroundings, the robot can effectively multitask, allowing it to execute various tasks simultaneously.
Efficient warehouse operations hinge on effective route planning, and integrating 360-degree barcode scanning capabilities ensures comprehensive coverage. This enables quick reads from any angle, ultimately minimizing the need for manual adjustments.
Understanding exact positions- pickup and placement
When a robot triggers a pick action in response to a corresponding request, it also simultaneously sends an image-capture request to each 3D camera. Through depth image analysis, the algorithm then precisely identifies the pick tote, updating the robot’s world model to ensure accurate positioning and prevent collisions while it performs a pick-action. Similarly, when items need to be placed into a tote, a new 3D image is generated to detect both the tote and its contents. A specialized stacking algorithm characterized by its intelligent design then determines the optimal stacking sequence, factoring in distance calculations to ensure gentle handling. In a way, it’s like playing Tetris— parcels of varying sizes and weights autonomously arranged for maximum space utilization and stability, without relying on predetermined stacking patterns.
Understanding the specifics of items- type, quantity, weight, dimensions etc.
2D cameras can be employed to identify items for picking, complemented by computer vision and deep learning algorithms to pinpoint their location and quantity within the tote. This information enables the robot to update its collision model and execute item retrieval without collisions. Additionally, the deep learning algorithms can also discern the material type of items; whether they are made of cardboard, paper, hard or soft plastic for example, enabling the robot to choose the correct type of suction cup to pick that material.
Meanwhile, a weight sensor integrated into the gripper can provide the mass of each item, helping in determining if the robot has accidentally picked up two items instead of one, and regulating its movement speed accordingly to prevent dropping them, especially if they are heavy. Given that pick totes may contain multiple items, potentially obstructing each other, the robot may initially lack precise awareness of all dimensions. This necessitates a new image of each picked item to accurately learn their dimensions. This task is facilitated by the 3D camera, which captures a fresh image of the item the robot is holding.
Determining the best approach
It’s also possible for the robot to ascertain the optimal grasp pose by precisely analyzing the orientation of the items. For instance, if an item is slanted, the robot may need to adjust its gripper to tilt its suction cup onto the correct surface.
Better vision = More reliability
Needless to say, improved vision and detection capabilities significantly boost reliability in robotic operations. Eliminating the need for laborious SKU teaching and fostering continual learning, the robot derives item information directly from the 3D images. The robot learns from each 3D image it analyzes. This enhances its proficiency, resulting in quicker and more accurate item detection. Thanks to the robot’s continuous monitoring of its environment, which includes the position of the pick and place totes and item dimensions, the likelihood of collision is also significantly reduced.
Promising developments in camera technology
The camera technology landscape is currently witnessing several interesting developments. Take the case of 2D cameras and you see a quick increase of resolution. In the realm of consumer cameras, it’s not uncommon to have resolutions of 12 to 48 MP for smartphone cameras, whereas in robotics, the resolution has often been limited to 1MP. But in recent years, suppliers are releasing resolutions of 5 MP, 8 MP and higher. It’s a welcome change, as now it’s possible to see smaller items and details a lot better.
Things are even more exciting for 3D cameras. If we look back ten years, there was very little supply (and demand) for 3D cameras. The available options were typically very low in resolution and unsurprisingly, inaccurate. Over the past year, however, there has been a notable increase in the number of suppliers offering 3D cameras with a range of technological specifications, significantly higher resolution, and consequently, improved accuracy. One particularly intriguing advancement in 3D camera technology is the integration of deep learning to enhance the quality of 3D data. Most 3D cameras find it hard to capture complete and accurate data for all surfaces within an image, often resulting in gaps or areas of high uncertainty. Now, the latest innovations involve cameras that utilize deep learning algorithms to intelligently fill in these gaps based on sample images.
A combination of vision, motion and task planning for enhancing robot reliability
Robots need to accurately handle a large variety of items and continuously adapt and improve efficiency, that too in complex environments. Achieving this requires a meticulously implemented orchestration of vision, motion and task planning. The integration of computer vision into robotics has revolutionized the way robots interpret their environments through digital images or videos, enabling object recognition, navigation and precise task execution. However, developing safe and efficient motion planning systems remains a challenge, requiring sophisticated algorithms to guide a robot's movements accurately.
Task planning and execution software further complements these systems, allowing robots to understand, sequence, and perform tasks autonomously. While motions make up the largest part of a robot’s cycle time, motion planning is used to direct the robot's path from A to B. Task planning, on the other hand, involves all actions a robot needs to execute, like move to pick position, create 3D image or turn gripper off, ensuring that it's carried out at the exact right time to reach its goal, for example, the picking and placing of an item.
About Heico Sandee
Smart Robotics Founder and CEO, Heico Sandee, holds a PhD degree and previously acted as program manager for robotics at Eindhoven University of Technology. With more than 15 years of experience in robotics development, Heico now leads Smart Robotics in developing intelligent, robot-independent software for flexible deployment of automated solutions.
About Smart Robotics
Smart Robotics is a specialist-developer of robotics and automated warehouse systems. The pick and place robotic solutions offered by the company are engineered to improve overall capacity, increase the reliability of warehouse operations, and tackle issues related to the continuing labor shortage in the logistics industry. These solutions are driven by Smart Robotics’ tech-trinity hardware and software. Smart Robotics’ tailormade automation solutions help improve working conditions for warehouse floor workers by taking over repetitive and physically strenuous tasks, such as order picking, packing, palletizing, and sorting.
The content & opinions in this article are the author’s and do not necessarily represent the views of ManufacturingTomorrow
Comments (0)
This post does not have any comments. Be the first to leave a comment below.
Featured Product
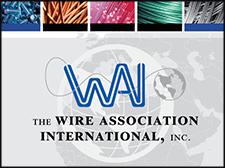