We still have a long way to go before we can truly enjoy the countless benefits AI has to offer. Not only that, but for AI and ML (machine learning) to gain real, feasible meaning in manufacturing and beyond, there are a few steps the industry needs to take.
The AI of the Future and Its Implications in Industrial Manufacturing
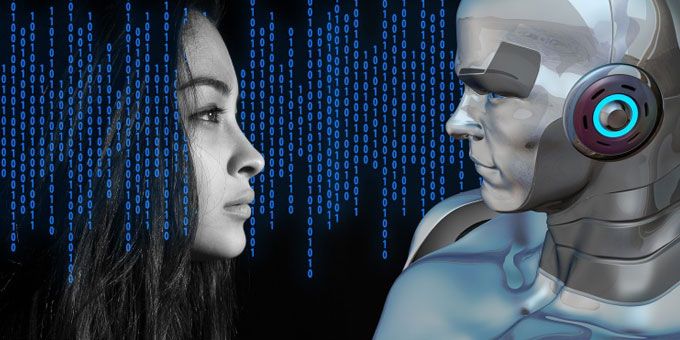
Avner Ben-Bassat | Plataine
Revolutionary technology tends to go through an interesting evolutionary process. At first, we’re just happy to learn about emerging technologies and excited to see them work at all; then, we’re curious to explore the many possibilities they have to offer; and finally, we want to put them to actual wide use and make the most out of it.
Artificial Intelligence and machine learning began as a science fiction-like notion, but soon became a part of every startup’s pitch deck. Fields such as image, speech and natural language processing have greatly advanced thanks to AI algorithms.
The manufacturing industry, specifically, has great faith and even greater hopes for what AI can do.
Is the AI promise fulfilled?
We still have a long way to go before we can truly enjoy the countless benefits AI has to offer. Not only that, but for AI and ML (machine learning) to gain real, feasible meaning in manufacturing and beyond, there are a few steps the industry needs to take.
As someone who has been practicing in this field for a few decades now, I would like to discuss some of the main limitations currently standing in the path of deep learning solutions, and propose actionable ways to address and solve them.
In other words, I would like to share with you my two cents re: what I believe should be the evolution of AI, and how it should improve in order to be able to fulfill its promise.
A learning experience: the limitations of deep learning
Collecting data is one thing, but putting it to good use is another. The following limitations explain what it takes for deep learning to become a working part of any industry toolbox:
- Lack of sufficient data: Every deep learning technology feeds off data. Lots and lots of data. In some cases, there’s simply not enough existing information to draw from. When one implements AI systems today, he or she may sit and wait for data to be collected by them. Precious time flies by before these systems become effective and have enough data to validate their processes. But who said it has to be that way? After all, data is constantly being processed by different platforms. Why can’t we lean on existing data?
- Non-transactional tasks: Non-transactional tasks require a stronger level of artificial intelligence capabilities, because they implement broader knowledge levels. This is the difference between sorting existing data and drawing conclusions beyond current information. AI algorithms today are limited by the conclusions that they can generate, which can’t be driven by existing information alone.
- Prediction and planning: Algorithms are challenged when asked to guess what’s next to come and how to prepare for it. This is one of the main capabilities we’d like to see AI achieve, and one of the hardest to conquer.
- Thinking vs. calculating: Sometimes, the benefit we’d like to achieve is not at all about calculating, but instead involves examining certain behaviors in a more outside-the-box, innovative manner. Newton’s realizations, for example, may have been mathematically proven, but they don’t necessarily stem from calculating. Some were born by observing and contemplating natural procedures. How can we teach an algorithm to do that?
- Glaring mistakes: We hear about funny mistakes made by AI software all the time, such as recommending a fast food place for Valentine’s dinner. And while that might be funny (though perhaps not for our date), serious businesses may incur real damages from obvious mistakes made by algorithms. Even if we reach 94% accuracy, the remaining 6% present us with enough to worry about, making it hard to fully trust a system.
- Explainable AI: One of the most difficult questions you can ask any AI-based technology is “why?” Developers often remain in the dark when it comes to the process behind certain decisions, and reasoning becomes a mystery that’s up for us to resolve.
- External knowledge: Should we offer additional information beyond the data provided to our deep learning technology? I believe that the existing knowledge surrounding us humans can and should be offered. Still, we need to decide when and how it should be implemented in order to develop our AI capabilities.
Double Deep Learning: The smart ‘Wikipedia’ concept
The first concept I’d like to raise is what is called ‘ReKopedia’ (reusable knowledge for smart machines, hence the name) by Dr. Moshe BenBassat. It relies on a simple logic: As humans, we take our natural intelligence and combine it with life experience and acquired knowledge, in order to make informed and (hopefully) better decisions. Why don’t we apply the same concept to deep learning by creating a massive knowledge base, sort of a Wikipedia of sorts, for smart machines to use in order to get more reliable information and predictions, as quickly as possible?
This would allow AI to draw from an existing, ever-expanding and constantly-updating pool of information that will improve many of the problems listed above.
ReKopedia can be an open-sourced database, offering the entire deep learning community an opportunity to receive and share data and knowledge that will speed up the value in AI solutions.
Specific industries will be able to form their very own knowledge-base, covering data events, predictions, technologies, business models, finance, marketing, and more. Smart machines will be accordingly taught to automatically convert this information into software structures that they can lean on.
Double up, please
But having a Wikipedia for machines isn’t enough on it’s own. Something else is needed.
Double deep learning combines data-focused techniques with deeper knowledge and reasoning. This second “deep” refers to “machine teachers” that focus on the fundamental principles of a certain field.
Instead of limiting deep learning to specific techniques, we should teach machines more complex theories and methodologies, and aim to go deeper in order to offer machine learning support for more perplexing situations.
Algorithms should be built on more than a need-to-know basis. It’s what they don’t necessarily know in the first place that will eventually help them complete the task.
Combining the two
For machine learning to take a leap forward, smart-machine Wikipedia and double deep learning should be implemented simultaneously. Together, the two present the connection between data and knowledge, calculating and thinking. We shall form a universal machine intelligence system that will scale faster and march AI forward.
But for the promise to be fulfilled, evolution must occur.
ABOUT PLATAINE: Plataine is the leading provider of Industrial IoT and AI-based optimization solutions for advanced manufacturing. Plataine’s solutions provide intelligent, connected Digital Assistants for production floor management and staff, empowering manufacturers to make optimized decisions in real-time, every time. Plataine’s patent-protected technologies are used by leading manufacturers worldwide, including Airbus, GE, Renault F1® Team, IAI, Triumph, General Atomics, TPI Composites, MT Aerospace, Kaman, Steelcase, and AAT Composites.
Plataine partners with Google Cloud, Siemens PLM, McKinsey & Company, TE W&C, Airborne, the AMRC with Boeing, and CTC GmbH (an Airbus Company), to advance the ‘Factory of the Future’ worldwide.
The content & opinions in this article are the author’s and do not necessarily represent the views of ManufacturingTomorrow
Comments (0)
This post does not have any comments. Be the first to leave a comment below.
Featured Product
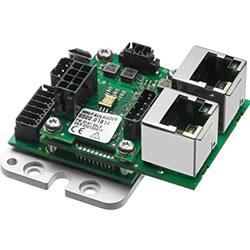