By tapping into existing maintenance or equipment logs, a manufacturer can apply machine learning to predict which connected devices or machines will be in need of servicing or forecast required inventory levels across warehouse locations...
Using IoT for Machine Learning-based Predictive Maintenance
Rohit Gupta | Sentenai
Please tell us a bit about Sentenai and their role in the manufacturing industry?
Sentenai provides infrastructure for automating data pipelines and makes it easier and more efficient to work with time series data. We allow manufacturers to collect data from either one machine or hundreds of sensors, logs and APIs, and then load that data into Sentenai without needing to predefine fields and types. We organize and optimize all data automatically so manufacturers can more easily derive insights from their existing machine data.
What's unique about manufacturing data/what makes it particularly difficult to leverage for predictive insights?
Most manufacturers are working with time-based or time series data, which can be really difficult to leverage from an organizational and formatting perspective. Also, considering the variety and number of different machines and sensors most manufacturers have in place, it can be tricky to combine all of those different data sources efficiently and in a manner that allows for predictive analytics.
For manufacturers without access to extensive data science teams, what can they do to really leverage their data to improve operational efficiency?
Seek out subject matter experts in your organization who know your machines’ behaviors better than anyone else. Work closely with them to determine what insights can be derived from your existing machine data and what real-world problems can be assisted or even solved by tapping into your data. Because at the end of the day, the important thing is not necessarily how much data you have to work with, but what productive questions can realistically be answered with your existing data.
How do you see machine learning and AI shaping how manufacturers use their existing data?
One of the most productive applications of machine learning and AI for manufacturers is predictive maintenance. By tapping into existing maintenance or equipment logs, a manufacturer can apply machine learning to predict which connected devices or machines will be in need of servicing or forecast required inventory levels across warehouse locations, thereby drastically decreasing downtime and waste. Machine learning allows manufacturers to determine the most effective questions to ask of their available datasets and continually grow and refine their predictive maintenance programs even as their business scales.
What are some of the biggest challenges these companies face in trying to do so?
Turning the idea of machine learning-based predictive maintenance into an actual deployment can be complex, however there are several best practices that can help drive results early in the process. For example, it’s helpful to start small in order to learn a repeatable process on a set of data focused on a singular use case. Also, deploy your machine learning models to a controlled group of machines first in order to validate the success of your models before putting them into production.
Why do businesses need to capitalize on their existing IoT data in order to remain relevant and competitive?
Any business that’s been collecting IoT or sensor data stands to gain incredible value from making that existing data actionable. Manufacturers fit this mold particularly well, as they already have so much IoT data and can use it to gain a competitive advantage by streamlining their operational processes, optimizing their demand forecasting and better understanding their customers’ propensity to buy. Leveraging their connected device data to build predictive maintenance programs can also lead to greater operational efficiencies for manufacturers.
How would you outline the road to successful data capture and integration for a midsize manufacturer wanting to incorporate it into their operations?
A crucial first step is to conduct a data audit. A data audit is a way to fully understand what data an organization or specific departments are generating and how that data can be leveraged into digital initiatives. The primary goal of a data audit is to fully document and understand the available data being used in each, individual business unit, and to perform a complete mapping of the data’s availability.
Once you’ve identified the available data being used as well as all data sources and any current workflows, it’s important to identify a specific use case that can drive business value and prove the efficacy of a data-driven approach. For instance, it might be helpful to start by understanding and predicting any instances of downtime at a single factory, or even on a single production line.
From there, agile reporting is key, as it provides continual, real-time visibility into machine data and access to forward-looking insights. Since many manufacturers have multiple data sources (like ERP, CRM, maintenance logs and IoT), agile reporting can be even more valuable as those different data sources can be fused together to pinpoint correlations between departments, processes and/or events.
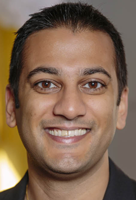
Rohit Gupta is the co-founder and CEO of Sentenai, an emerging data science technology company. Rohit has been on both the investing and operating side focused on software infrastructure related companies. He previously served as a Director at Techstars Boston, where he helped select, invest and mentor early-stage startups. He holds a BS in EE from MIT.
The content & opinions in this article are the author’s and do not necessarily represent the views of ManufacturingTomorrow
Comments (0)
This post does not have any comments. Be the first to leave a comment below.
Featured Product
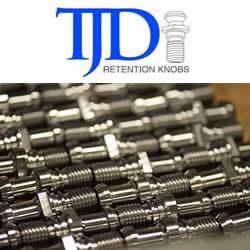