Q&A with Ravi Kunju, Altair: Digital twins offer designers the opportunity to analyze the impact of operational conditions without requiring the production of a prototype until much further along in the manufacturing process when mfg's have a greater certainty of success.
Simulation Design for Rapid Prototyping
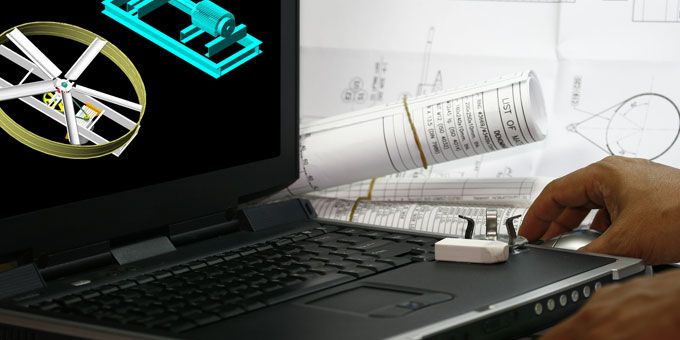
Ravi Kunju | Altair
Tell us a bit about Altair and your role in the advanced manufacturing industry?
Altair is a global provider of design engineering software and cloud solutions for product development, high-performance computing (HPC) and data intelligence. Through Altair’s simulation platforms, organizations can apply advanced numerical methods to optimize and transform the way they design products by being cognizant of both performance and manufacturing, for improved performance at an accelerated time to market, and at much lower costs.
As more organizations look to additive manufacturing tools such as 3D printing for lightweighting and part consolidation as part of their digital transformation strategies, Altair’s simulation-driven design technology integrates data analytics and true-to-life visualization with design optimization to significantly enhance today’s newer rapid prototyping methods.
How would you describe rapid prototyping? Why has this term been getting so much attention lately?
The formal definition of rapid prototyping is described as a group of techniques used to fabricate a scale model of a physical part or assembly using 3D CAD data. The main purpose is to verify if a proposed idea or design meets the aesthetic and performance requirements before mass or actual production. Much of what we are doing at Altair with simulation technology is virtual prototyping. Applying advanced physics and optimization methods, and combining them with high-performance computing allows us to actively aid in the simulation-driven design process, limiting the need for making multiple physical prototypes.
Our customers have relied on simulation technology to make earlier design and engineering decisions and optimize both their designs and their tools, improving their product lifecycle management process and thus moving towards more rapid “digital” prototypes (Reference: https://www.spotlightmetal.com/how-simulations-drastically-reduce-the-development-time-of-cars-a-797868/).
Broadly, rapid prototyping can be classified into two categories: digital and physical.
Rapid prototyping is becoming an increasingly growing trend as more and more organizations look to leverage new technologies like 3D printing that help them deliver products to market even quicker for competitive advantage. Technology advancements over the last 20 years have made this possible. Interestingly, the freedom that 3D printing offers in creating radically new shapes marries the advancements in both ‘digital’ and ‘physical’ prototyping into a powerhouse of possibilities. That is the reason why we see so much interest in rapid prototyping today.
Prototyping is known for being expensive both in terms of time and costs. How much confidence do manufacturers have in simulation today to minimize the number of prototypes or even replace prototyping?
Physical prototyping is not going away. There is still a lot of need for it, such as with regulatory and certification purposes. However, due to an increased level of confidence in virtual simulation methods over the years, the need for making multiple physical prototypes has reduced significantly.
With advancements in topology optimization technology, we’ve moved from CAD being a driver of design, to virtual simulation driving the design. For example, by utilizing topology optimization methods we can look at the requirements for performance and manufacturing simultaneously and let the software virtually grow the model for the ideal outcome for maximizing efficiency.
There are, of course, different expectations for each industry, and an opportunity to apply new technologies to cut down on these design lifecycles. For instance, the product design lifecycle for an aircraft can be up to 10 years, a car can be 18-24 months, and a cellphone can be about 6 months. By combining the best methods of digital and physical prototyping we have the potential to cut these timeframes in half.
How are new technologies like 3D printing shaping the prototype industry, including speed to market, quality, and accuracy of designs and product lifecycle?
When it comes to the production of customized single-unit parts, innovative solutions such as 3D printing offer customers higher productivity at dramatically lower costs compared to standard solutions.
For example, in the plastic injection molding industry, one of the most important parameters for higher productivity is the cycle time to produce a single part, which can be optimized through conformal cooling leading to faster cooling time. This process also reduces the thermal deviation in a mold, leading to better component quality and shorter cycle times. However conformal cooling lines are hard to fabricate by conventional machining. By applying simulation technology to toolmaking applications, manufacturers can 3D print structurally lightweight designs that are customized to their tool molds with conformal cooling. This helps in quickly producing parts that are less expensive and better (and in some cases, previously impossible) than those created with traditional methods. These are the first cases where the techniques developed for rapid prototyping methods are well on their way to be established as viable methods for efficient and economical mass production.
At what stage will new additive manufacturing technologies become mainstream, moving from prototype to production and gain the same level of trust as other traditional methods?
As discussed previously, the synergies between ‘digital’ and ‘physical’ prototyping methods are transforming mainstream production with additive manufacturing (AM) being the bridge between small and large batch production. However, the challenge is predicting the behavior of the material after manufacturing. In traditional manufacturing like casting, forming or forging, the industry has 100 years of trust in what is viable to make sound engineering judgment. AM does not have that luxury today, as it is rapidly changing and is relatively new.
Selective laser melting has been the most popular for metal 3D printing, however, due to the low speed, high cost and size restrictions coupled with inconsistent material properties due to gas entrapment and process variability, it is extremely hard to categorically accept it as a viable mass manufacturing method today. However, for low volumes and highly complex structures that are relatively small, it is surely becoming mainstream. Today the dental industry is extremely mature in using 3D printing. A dentist can take a scan and get a 3D printed crown in a couple of days. The entire process is completely automated. We are also seeing similar applications in personalized foot inserts in plastics where Fused Deposition Modeling (FDM) and binder jetting is used. The materials used for FDM have increased in number over the last few years, including biocompatible products with good mechanical properties.
Metal binder jetting is another new method that has the potential of taking the additive manufacturing industry into serial production. Similar to traditional paper printing binder jetting uses layers of powder and binder (like glue or ink) and forms a loosely bound part called a green part that is sintered (heat-treated) to produce the final product. Due to its ability to produce solid layers at very high speed, binder jetting is being considered as a better option for 3D high volume printing.
Wire arc additive manufacturing blends traditional welding with robotics to produce very large scale parts. Although the process can be slow and might require machining for surface finish, the physics is more familiar and as such more reliable in trusting the properties of the final part. New methods in AM are emerging rapidly and our mission is to make sure we understand the subtle requirements so we can steer and numerically prescribe the most efficient designs.
At some point, we’ll start to see an increase in trusting the process. As mentioned earlier, with injection molding of dyes and metals in a hybrid approach where AM is being used in conjunction with traditional manufacturing. The insights we are latterly gaining from data analytics can accelerate the process of better understanding cause and effects in the complex AM processes to go mainstream; which is likely to happen sooner than we expect, but later than we actually want.
How does the knowledge gained (data insights) from simulation design technology and physical prototyping come together to influence the entire supply chain?
Rapid ‘digital’ prototyping, taking shape in more traditional manufacturing processes, is through the application of completely virtual, digital twins integrated into simulation design. Digital twins offer designers the opportunity to analyze the impact of operational conditions without requiring the production of a prototype until much further along in the manufacturing process when manufacturers have a greater certainty of success. In other words, you don’t need to crash test as many prototype cars before arriving at your end result, the final production vehicle.
As the volume of physical, outside data accrues from not only historical data, but through analytics and real-time data acquired during the complete design cycle, we anticipate that the entire supply chain will start applying machine learning to better connect cause and effects. This will be applied to production decisions in the same way as other manufacturing businesses. This technique will ensure that early design direction aligns with part yield, repeatability, cycle time, production planning goals and reduced costs.
In this context, the greatest promise comes from testing protocols that integrate digital twin technology with existing machine learning (ML) programs receiving streaming data via outside sources such as the Internet of Things (IoT). This is when the data is valuable not only for streamlining the design cycle but also for manufacturing the product since we’re creating a foolproof design that won’t fail in the plant. At Altair, we provide a complete, open and flexible platform for digital twins that are physics-driven and/or data-driven. By connecting insights from the virtual model and applying ML to the physical model, we’re closing the loop and optimizing product performance throughout its entire lifecycle.
How are manufacturers using data science to improve the product design lifecycle, including applying insights to topology optimization and/or generative design?
In the process of driving the design, it’s important to understand the actual quantitative performance requirements and the manufacturing constraints. Sometimes it’s easy to understand the constraints but other times it’s not, as there may be multiple variables that can influence the outcome.
When you are testing a machine prototype and something breaks down, there is a lot we can look at from a historical point of view: How often does this happen? Or is it with one particular supplier? Is it due to temperature, and on a particular day? Or is it the combination of all?
These are things you can look at mathematically, but it’s much more challenging to factor this into new designs, especially as outside variables are constantly changing. You really need the data step-by-step to learn and apply this to the design during the manufacturing process. This is where data science, including insights gathered from ML and IoT, is applied to the design in real-time (as with the digital twins), leading to real value.
When designing a next-generation passenger car, for example, engineers would receive real-time operational data from an actual vehicle including its speed, external wind speed, the temperature inside the cabin, the outside temperature, the rate of fuel burn, etc. That data would be fed to the digital twin, which would analyze how these conditions affect each other (i.e. How does fuel burn affect weight?) and how design changes could affect these conditions: What would be the impact of changing the external shape design? How can we improve the air conditioning system or vibration or acoustics characteristics? Real-time, real-world measurements would continuously enhance the simulation model.
Few manufacturers have integrated these existing technologies and applied them to their simulation processes. When they apply ML, IoT, and digital twins in a coherent, integrated way they will significantly improve their new product lifecycles by speeding time to market, reducing design, testing, and production costs, and creating more innovative products that better serve the needs of their customers.
Additive manufacturing is new and complex. Capturing and relying on physics to be absolutely accurate in a reasonable time can be challenging using simulation methods and compute resources available. Engineering a solution in these cases is extremely important, and machine learning can immensely help in connecting the dots between cause and effect. In selective laser melting, for example, calibrating a new powder requires fine-tuning 100s of parameters: laser wattage, speed, hatch spacing, direction, and cooling cycles to produce one good part. ML can immensely help here as mentioned above.
Where do you see the biggest advancements happening in simulation design and rapid prototyping in the next 5-10 years?
We see rapid advancement in both ‘physical’ (3D printing) and ‘digital’ prototyping (virtual simulation), but there will be a catch-up battle between the two. Both are severely intertwined; if one cannot capture issues virtually upfront and trust the final quality and results from the digital prototypes, the physical methods are going to have limited acceptance. On the other hand, the interest in software vendors to capture the physics is based on the viability of the method and its wide acceptance. Manufacturers, academia and research institutes all have to come together because as long as specific processes are not economical, they will rarely be touched by large OEMs. They will only be pursued by the bold ones who are willing to take risks.
We do see hybrid methods applicable in the injection molding, die casting and sand binder jetting for making sand cast and cores. 3D printed molds, dies, and patterns are being used more in conjunction with traditional manufacturing.
The urge for increasing the volume of production is making new methods like metal binder jetting interesting. Better prediction of material shrinkage during sintering and the post-manufacturing properties are being understood, and if successful, they can have a significant impact on large-scale adoption.
Better predictive capabilities combining ML and virtual prototyping also have the potential for large-scale acceptance of selective laser melting and powder bed 3D printing.
About Ravi Kunju
Ravi Kunju is Senior Vice President, Business Development & Strategy – Simulation-Driven Design at Altair. Mr. Kunju has been active in the area of advanced engineering analytics for over 20 years, promoting and delivering lectures on advanced simulation methods around the world. His publications have covered the application of unique numerical optimization methods to solve manufacturing problems. He earned a master's degree in mechanical engineering from Wayne State University, and an MBA from the University of Michigan, Ann Arbor.
The content & opinions in this article are the author’s and do not necessarily represent the views of ManufacturingTomorrow
Comments (0)
This post does not have any comments. Be the first to leave a comment below.
Featured Product
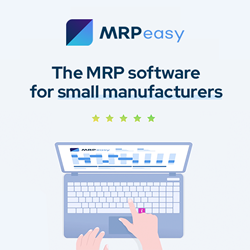