The Future of AI in Warehouse Design
Maintaining the seamless flow of goods and products is essential for success in the ever-evolving world of material handling. From manufacturing plants to distribution centers, the efficient handling of materials is not only a logistical challenge but also a critical determinant of competitiveness and profitability for organizations. In this fast-paced environment—where margins for error are razor-thin and demands are ever-increasing—the integration of advanced technologies has become imperative to stay ahead of the curve.
Artificial intelligence stands at the forefront of this technological revolution. Everyone is talking about it and wondering how it can and will help their organization. At its core, AI offers a collection of tools and methodologies that empower machines to learn, adapt and make decisions without human intervention. As industries embrace the potential of AI to optimize operations, the realm of material handling emerges as a prime candidate for transformative innovation. Guided by the insights of Andrew Ng, a leading AI expert and founder of Deep Learning.AI, we can explore how AI is reshaping the future of material handling, offering a glimpse into a world where efficiency, precision and innovation converge to achieve unparalleled levels of performance and productivity.
From supervised learning algorithms that enhance quality control processes to generative AI models that revolutionize warehouse layout design, the journey into the intersection of AI and material handling promises to unlock new frontiers of efficiency and effectiveness, envisioning a future where the seamless integration of human ingenuity and machine intelligence redefines what is possible in the industry.
Harnessing AI for Efficiency and Innovation
When it comes to warehouse design, AI's diverse capabilities offer a variety of opportunities to optimize space utilization, streamline workflows and enhance operational efficiency. According to Ng, AI can be categorized into four fundamental types, each offering distinct advantages and opportunities for optimization: supervised learning, unsupervised learning, generative AI and reinforcement learning. Each type builds off one another and allows AI to be increasingly more independent—to think, act and create on its own.
- Supervised Learning: This foundational approach to AI involves training algorithms with labeled data, enabling them to recognize patterns and make predictions. Supervised learning is akin to quality control processes in material handling, where machines learn to distinguish between acceptable and defective products. Vision systems, equipped with supervised learning algorithms, play a crucial role in identifying inconsistencies in production lines and streamlining inspection procedures.
- Unsupervised Learning: Unlike supervised learning, unsupervised learning algorithms operate without labeled data, allowing them to uncover hidden patterns and structures within vast datasets. For instance, in a notable project led by Ng, unsupervised learning algorithms sifted through millions of YouTube videos to identify everyday objects. Without training it or teaching it anything, the AI was able to pull out similar objects in the videos, which happened to be cats. This unlocked a promising tool for people to use, especially in material handling, where it can be used to optimize inventory management and forecast demand based on historical data patterns.
- Generative AI: The highly anticipated launch of OpenAI’s Chat GPT unleashed a wave of creativity and innovation across industries. By analyzing extensive datasets, generative AI algorithms can autonomously produce new content, designs, and solutions. For example, when you type something into Google’s search bar, it predicts the next word. In material handling, generative AI facilitates the rapid iteration and optimization of warehouse layouts, enhancing space utilization and operational efficiency. In tasks requiring AI to comprehend human language and convert it into computer code, such as inventory management and order processing, generative AI streamlines workflows and accelerates decision-making processes.
- Reinforcement Learning: Inspired by human learning processes, reinforcement learning algorithms improve performance through trial, error and feedback mechanisms. In material handling, robots with reinforcement learning algorithms can adapt and optimize their actions in dynamic environments. For instance, robotic arms learn to grasp objects more effectively by refining their movements based on feedback from previous attempts. This adaptive capability enhances the reliability and efficiency of automated material handling systems.
The Next Frontier in Material Handling
While current AI applications are largely specialized, ongoing research aims to develop AGI, which is capable of performing a wide range of tasks with human-like versatility. AGI represents the culmination of integrating diverse AI toolsets into a unified, adaptable system. It holds the potential to revolutionize supply chain management by easily orchestrating complex operations and decision-making processes.
By leveraging AI's evolving capabilities, the material handling design industry can benefit in several impactful ways:
- Personalized design suggestions: In addition to analyzing individual preferences, AI algorithms could also analyze data on broader trends and styles to provide insights into popular design choices, helping stay ahead of the curve and offer clients innovative and trendy options.
- Automated product suggestions: AI algorithms could use data on past-use items and preferences to suggest products that are likely to be of interest, possibly reducing design time.
- Design automation: AI algorithms could also suggest furniture, racking, floor layouts and more to be optimized for function and flow, considering factors such as movement patterns and usability.
- Natural language processing: In addition to improving efficiency and ease of use, natural language processing could assist with language barriers or disabilities, allowing more individuals to participate in the design process.
Tools such as CET Material Handling set new standards in material handling system design. Innovative software is a game-changer for intralogistics, warehousing and distribution center development, as users benefit from tailored solutions that enhance accuracy and efficiency. CET operates seamlessly in both 2D and 3D and utilizes Parametric Graphical Configuration (PGC) technology to eliminate errors and streamline the design process. Real-time Bill of Materials and pricing, along with comprehensive documentation creation, contribute to error reduction and overall project success.
The Era of AI
The convergence of AI and material handling systems promises a new era of efficiency, innovation and adaptability. By leveraging supervised, unsupervised, generative AI and reinforcement learning, industries can optimize their processes, enhance productivity and stay ahead in an increasingly competitive marketplace. Harnessing these capabilities allows warehouse designers to create spaces that are not only optimized for efficiency but also agile and adaptable to evolving operational needs, promising a future where machines and humans collaborate to unlock new frontiers of efficiency, productivity and supply chain stability.
About the author:
Rich Trahey has more than 25 years of experience within the production automation industry. Today, he and his team are connecting manufacturers and solution designers to find a way forward for more efficient and sustainable growth using Configura’s intelligent platform and growing ecosystem. Outside of work, Rich enjoys spending time outdoors with his wife and two kids in West Michigan or lacing up his sneakers to hit the basketball court.
Comments (0)
This post does not have any comments. Be the first to leave a comment below.
Featured Product
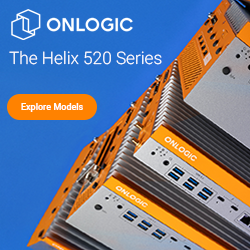