The battery pack cost of $100/kWh is a primary target for Tesla. To achieve this, the company must solve one of the world's most demanding technology challenges – and it is how to increase the volumetric energy density of battery cells while slashing production costs.
Could Predictive Analytics Help Tesla Drop the Cost of the Battery Pack to $100 per kWh?
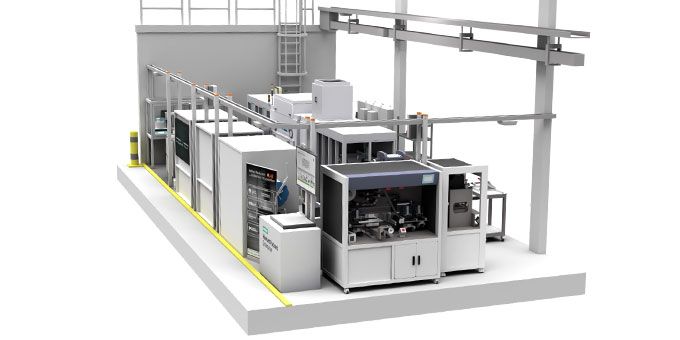
Kari Terho, Director of Smart Factory | Elisa Corporation
“Yes,” says Kari Terho, General Manager of Elisa Smart Factory. He explains in this article how his team has boosted the battery cell production yield by 16% with Predictive Quality Analytics at eLab, the electromobility research center in the University of Aachen in Germany.
The battery pack cost of $100/kWh is a primary target for Tesla. To achieve this, the company must solve one of the world's most demanding technology challenges – and it is how to increase the volumetric energy density of battery cells while slashing production costs.
When the founder of Tesla, Elon Musk, spoke in public on the company’s earnings call in 2017, he asked, “Can someone please come up with a battery breakthrough? We’d love it!” His sentiments reflect the complexity of the challenge that his company tries to solve.
To address Musk’s request for battery breakthrough innovations, this is how Elisa’s data scientists have increased battery cell production yield by predicting manufacturing quality!
There Is A Quality Challenge in Battery Cell Manufacturing
The manufacturing of Lithium-Ion battery cells for electric cars is, in principle, a straightforward process.
Anode and cathode electrodes are produced in several different sub-processes from a mixture of different raw materials and then packaged into battery cells, which are filled with the electrolyte, which is sealed and finalized before being sent-off to the end-of-line testing.
The process is, however, much more complicated than that. A primary challenge in the manufacturing of battery cells is that the final quality of the manufactured cells can only be verified after lengthy end-of-line testing – and completing the test can take up to three weeks.
It is only after this point when the manufacturer can determine if a final battery cell product can be used further, for battery pack production; or if it has to be scrapped as hazardous waste.
Scrapped battery cells cannot be recycled, and this results in scarce, non-renewable and expensive raw materials such as lithium, cobalt, nickel sulfate, copper, aluminium, and graphite being wasted in the process.
The global average first-time-yield (FTY) for battery cells is estimated to be as high as 15 percent, which makes battery cell manufacturing costly and slow.
How Can We Increase Battery Cell Manufacturing Yield?
At eLab, the electromobility research center in the University of Aachen in Germany, this quality challenge was identified as a significant issue which prohibits cost-efficient manufacturing and slows down the adoption of environmentally-friendly electric cars.
On the quest of developing a more efficient manufacturing process for battery cells, eLab partnered with Elisa Smart Factory’s data scientist team.
Using Predictive Quality Analytics For Battery Cell Manufacturing
The Predictive Quality Analytics is used to extract data from a manufacturing process to determine data patterns, based on which quality-related trends and outcomes, can be predicted. Hence, it is an appropriate tool to solve eLab’s quality challenge!
Elisa’s data scientist team followed the cross-industry standard process for data mining, the six-step CRISP-DM process; which is the most widely-used analytics model among data mining experts.
Here is how the process is outlined.
- The first step is to form a clear business understanding of battery cell production and to set the right goals. The overall situation was assessed, the quality drivers in the process were defined, the data points affecting the battery cell quality were identified, and the parameters, which best describe battery cell quality, were determined.
- The next step is data understanding, as in the CRIP-DM process. It involved a gap-analysis of what kind of data is available vs. what data is required. In this case, a data gap was covered by installing a quality camera on the manufacturing line.
- In the data preparation phase, the data is cleaned and harmonized into the same format. Timestamps are cross-checked to avoid data collection losses.
- The modeling was the most challenging phase for the data scientists. There are thousands of ways to analyze data. The data scientists must try out different algorithms to see the various outcomes they give when applied to the data collected from the battery cell manufacturing line.
- The evaluation of results is critical. It involved investigating if the results are valid and if they enable predicting the quality of the battery cells.
- Finally, at the deployment phase, the team defined the optimal parameters for setting up the production equipment and machines for optimal production quality. This included the right viscosity parameters, among many others.
Result: A16 percent Increase in Battery Cell Production Yield!
After Elisa’s Predictive Quality Analytics was deployed, the scrap rate at the eLab battery cell production line dropped by 16 percent, as the quality of the battery cells could now be predicted early in the process. The battery cells predicted to be of sub-standard quality at the end of the process are now identified earlier in the process and taken aside from the line. The raw material could be recycled for a new production batch, instead of being scrapped into non-recyclable waste, after the end-of-line test three weeks later.
In Conclusion
What would be Tesla’s price tag be for a production yield gain of 16 percent? Hard to say, but imagine this…
If the entire production capacity of 23 GWh at the Tesla Gigafactory 1 was solely engaged with the production of the 2170 battery cells of Tesla Model 3 car, its annual output could be 1.3 billion units of the 2170 cells. The 16 percent yield gain would correspond to over 200 million more cell units produced – that’s enough for 49,000 Tesla Model 3 Long-range cars!
Imagine, a cost-saving of $ 400 million could be achieved, if the battery cell production cost of $ 111 per kWh, as estimated by the UBS bank, is assumed.
Regardless of the impact on any car manufacturer’s business, the electric vehicle industry must continuously evolve its manufacturing practices and efficiencies. Lithium-ion batteries are made of rare earth minerals like cobalt, lithium, nickel, and other metals, which should be used efficiently to feed the rapidly growing demand for electric cars!
About Kari Terho
Kari Terho is the Director and Head of Smart Factory at Elisa Corporation, the leading ICT service provider in Finland. Prior to joining Elisa Smart Factory, Kari held various leadership positions in service management, and sales and business development at tier-one wireless service providers, and at global blue-chips including Hewlett-Packard. Kari holds an MBA in Business and Administration.
About Elisa Smart Factory
Elisa Smart Factory is a leading provider of artificial intelligence and industrial IoT software for industry manufacturers. We connect to any data source, harness streams of data, and combine data analytics and machine learning to create outcomes like increased uptime, production quality and yield.
The content & opinions in this article are the author’s and do not necessarily represent the views of ManufacturingTomorrow
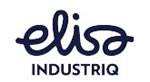
Elisa IndustrIQ
Elisa IndustrIQ is an industrial manufacturing software provider enabling manufacturers to translate data into actionable insights. This supports customers to achieve flexibility, resilience, and clarity throughout the entire supply chain. Additionally, Elisa IndustrIQ is one of the frontrunners of implementing AI and advanced cybersecurity into manufacturing software solutions, helping manufacturing companies achieve a sustainable future through digitalization.
Other Articles
The AI Imperative: Crafting Your Manufacturing Future
Human-Centred Digitisation: Bridging the Gap Between Technology and People in Manufacturing
Cybersecurity in Manufacturing: From the Shopfloor to Online Safeguards
More about Elisa IndustrIQ
Comments (0)
This post does not have any comments. Be the first to leave a comment below.
Featured Product
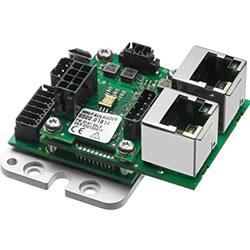