In contrast to traditional corrective maintenance or scheduled maintenance, CBM is an approach whereby maintenance is performed proactively on evidence of need identified through direct or indirect monitoring and predictive analytics of the assets of interest.
Condition Based Maintenance (CBM)
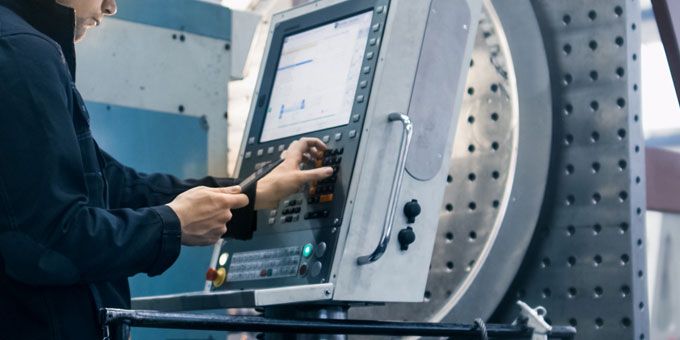
Kai Goebel | PARC
Please tell us a bit about PARC and your role in the advanced manufacturing industry?
The Palo Alto Research Center (PARC) was founded in 1970 as Xerox PARC with an initial charter to create “the office of the future”. Early work focused on how to modulate a laser to create a bit-mapped electronic image on a xerographic copier drum that led to the invention of the laser printer. Shortly thereafter, the Xerox Alto workstation was created which employed the world’s first bit-mapped display, graphical user interface with windows and icons, local area network, a mouse, and other groundbreaking personal computing innovations. Fast forwarding 40 years, PARC is now an independent company (owned by Xerox) and explores innovation in a number of competencies, one of which is focused on digital design and manufacturing. Specifically, PARC is creating a new science of computational design by inventing new representations and algorithms to design, analyze and plan the manufacture of highly complex structures that can be fabricated with state-of-the-art technology. This interdisciplinary work unifies principles of computational physics, geometric reasoning, automated spatial planning, and field modeling to help engineers create entirely new kinds of products – the likes of which many have not yet imagined. In parallel, PARC has spearheaded the development of CBM techniques (called MOXI) which allows for online anomaly detection, diagnostics, and prognostics of industrial assets towards actionable predictive maintenance. These techniques have been customized and successfully deployed it in many challenging customer environments, including manufacturing floors of major global organizations.
How do you define Condition Based Maintenance (CBM)?
In contrast to traditional corrective maintenance or scheduled maintenance, CBM is an approach whereby maintenance is performed proactively on evidence of need identified through direct or indirect monitoring and predictive analytics of the assets of interest. To that end, specific knowledge of the asset’s condition is obtained by analyzing sensor information that allows assessing the asset’s condition at any given time in its operating life. Maintenance action can then be planned with sufficient lead time to minimize the cost and operational impact of the occurrence of a failure. CBM differs from “on-condition” maintenance in that there is an understanding of how much time is available before the required maintenance must be performed.
How should one go about deciding whether CBM is right for my company?
The first step is to get a good understanding on the effect of equipment downtime on operations by quantifying the impact and juxtaposing that with the cost of implementing CBM. A cost-benefit analysis will then help to decide whether this makes sense and for which equipment it might make sense. One would start by first methodically adding up the value lost due to downtime, the cost of repair, logistics, warranty claims, time/cost for part replacement and inventory, etc. On the cost side, there is some hardware and software development cost, and cost for maintaining the infrastructure. One can dig further down to factor in secondary cost and value elements but it is good to start at a high level to get a rough idea whether there is an opportunity for savings.
What are the hurdles of implementing CBM?
One may have to face technical and non-technical hurdles. On the technical side, it is required to have some insights into assets’ health via sensor measurements and relevant system information/details. While the IIoT, smart maintenance initiatives, and industry 4.0 have been receiving a lot of buzz, having the right kind of sensors deployed at the right locations of the equipment and collecting and managing the data are still hurdles that need to be overcome for many companies. It would be desirable to do some upfront analysis of fault modes (maybe by conducting a Failure Mode Effect and Criticality Analysis) to understand which sensors ought to be deployed where. It is also critical to leverage the right kind of analysis tools that can reliably provide information about anomalies, fault root causes, and remaining life at the desired accuracy levels without triggering undue false alarms or missing key events.
On the non-technical side, it is critical to have a champion within the organization who has budget authority to make needed investments. Just as important is it to gain trust of stakeholders so they understand that the technology is not a threat, but a benefit to how they conduct business.
What are the benefits?
If done right, CBM can help to stretch maintenance budgets by proactively focusing action on assets in need; reduce downtime by preventing failure, thereby increasing availability; reducing risk of loss of system, and increasing safety; improving spares/personnel management and better inventory control; providing better diagnosis and fault isolation through root cause analysis, thereby decreasing troubleshooting time; reduction in take-back cost and disposal avoidance; In the long run, it can also enable better asset/system planning, and vital operational feedback for design and stakeholder teams.
There is a lot of hype surrounding machine learning and AI in the context of CBM. What expectations should a company have with regards to these technologies?
Yes, we see a lot of promises being made that all maintenance woes can be solved by simply sprinkling some “deep learning” or other popular machine learning technique over operational data without more insight into the manufacturing environment. As is the case with all machine learning approaches, one needs to have access to a large number of relevant data to train the models. For CBM, “relevant data” means many observed trajectories of sensor measurements over experienced faults in the field. These have most of the times actually not yet been experienced. Some types of equipment do not frequently fail with the same fault mode. Even if they do, the data have historically not been collected for all operational modes. Therefore, the performance of many of these techniques will likely disappoint. It would be advisable to be clear-sighted about where and how AI techniques can augment CBM and where physics-based models have the upper hand – or combine the two similar to what we have done effectively in MOXI for several clients and critical systems. And, of course, a sound understanding of the underlying engineering processes and tight integration with the manufacturing floor are paramount.
The content & opinions in this article are the author’s and do not necessarily represent the views of ManufacturingTomorrow
Comments (0)
This post does not have any comments. Be the first to leave a comment below.
Featured Product
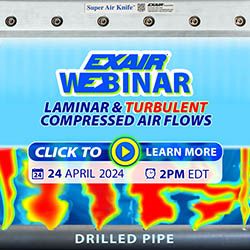