The Future of IoT Analytics: How AI and Big Data are Changing the Industrial Sector
The Internet of Things (IoT), a technology based on the concept of connecting physical objects to the Internet in order to generate real-time data, is changing the way the industrial sector collects and processes data.
Imagine trying to keep track of everything happening in a giant factory, where robots build cars, sensors check the temperature, and cameras inspect for defects. Without a system to connect all that information, it would be chaotic.
With over 22.4 billion IoT-connected devices expected by 2026, manufacturers are transforming into data-driven powerhouses. It's why forward-thinking companies like GE and Siemens are leading the way in developing sophisticated ecosystems in their manufacturing plants to integrate input sources to collect massive amounts of data.
The large amount of data collected from IoT inputs creates both advantages and challenges. In order to use this data effectively, organizations have to employ artificial intelligence (AI) and machine learning (ML). These technologies are making it possible to do real-time analysis, maintenance, and fault finding by outputting actionable intelligence. In my experience working across large-scale data and AI systems at Cisco, AT&T, and Walmart, I’ve seen how the integration of AI, machine learning (ML), and big data platforms is enabling organizations to move from reactive maintenance to predictive intelligence.
In my own work in large-scale telecom environments, The ML-driven platforms I developed processed high-velocity sensor data to identify degradation trends and usage anomalies. This helped operations teams predict failures in advance and take proactive steps—illustrating how predictive maintenance minimizes both cost and service disruption.
The conventional approaches to maintenance are either through planned management or through reactive corrective maintenance, both of which result in production downtime and high costs. The use of artificial intelligence in industrial predictive maintenance models includes the use of different sensors that include temperature sensors, vibration sensors, and pressure sensors to identify the signs that a machine is on the precipice of failure. Predictive maintenance uses IoT sensor data to tell when equipment is likely to fail, giving companies a chance to act before the failure occurs, and allows them to schedule repairs during optimal times.
At Cisco, where I led the architecture and implementation of a real-time usage analytics engine within the IoT business unit, the engine processed telemetry from connected devices and delivered hourly and daily insights, which was previously not possible at this scale (200M devices and 30B data traffic records per day). More notably, I developed and deployed distributed anomaly detection models that could catch subtle deviations in sensor behavior—crucial for preventing system failures in time-critical environments.
A great example of that is in the aviation sector, where predictive maintenance can be used to check the health of the engines to ensure that they are repaired or replaced only when they are worn out and not based on a time frame. This approach not only maintains the lifespan of the equipment, but also reduces the frequency of unexpected breakdowns and the resulting effects on service delivery.
Edge Computing: Real-Time Intelligence at the Source
While the AI and ML conventional cloud-based data analysis systems work for many companies, they are often ill-suited for many band- and latency-constrained IoT applications typical of industrial environments. Edge computing, which is the processing of data at the edge of the network, that is, not in the central cloud, is quickly becoming one of the most important emerging technological solutions.
In practice, I’ve worked on implementing edge-based anomaly detection and self-healing models for distributed IoT systems. These models allowed edge devices to autonomously identify issues and recover from them without cloud dependency—significantly improving response times and system resilience.
Moreover, edge computing offloads the burden from cloud infrastructure, cutting down on the cost of operation. Nimble companies can select the data to be sent to the cloud, while at the edge, they can select the data to be kept or discarded. This hybrid approach offers faster response time, increased reliability, and improved security through the minimization of risk of being vulnerable to cyber-attacks.
When companies implement AI for data unification, they are able to get an overall view of their business, improve the communication between the systems, increase efficiency, and spot problems before they arise.
I’ve seen this first hand in large-scale retail and supply chain ecosystems, where AI-driven models unified data across fulfillment systems, in-store operations, and inventory management. This not only improved forecasting accuracy but also enabled real-time anomaly detection in product movement and customer behavior.
In big industrial systems, unexpected issues—called anomalies—happen all the time. Anomalies are common occurrences in industrial IoT environments and they are crucial for monitoring for exceptions, security alerts, and maintaining overall system health. These could be errors in machinery, security threats, or warning signs that something is about to break. Older methods of detecting these issues often miss important details or give too many false alarms. That’s where AI and machine learning come in. Instead of just following set rules, AI learns what "normal" looks like for a system and then notices when something is off in real time.
Power grids use AI to monitor electricity flow. If the system detects unusual voltage spikes, it could mean a piece of equipment is failing—or even that hackers are trying to mess with the system. Catching these problems early prevents major outages. AI-powered anomaly detection is also crucial in healthcare, where smart medical devices need to be extremely reliable. By learning from past data, AI can tell the difference between real threats and harmless glitches, making sure hospitals and doctors get accurate alerts when something needs attention.
AI and ML are defining the future of IoT analytics in the industrial sector. By providing real-time analysis, maintenance, and anomaly detection, edge computing architectures guarantee that data is processed properly, and PM optimizes equipment conditions and saves costs.
Across different sectors—ranging from telecom to IoT infrastructure to retail—I’ve experienced how scalable AI and data architectures empower real-time decision-making, reduce friction, and prepare organizations for the next wave of industrial intelligence.
As the technology matures, these systems will become even more intelligent and predictive. Companies that invest today are not just upgrading infrastructure—they’re future-proofing their operations for the age of intelligent industry.
Nirup Kumar Reddy Pothireddy is a seasoned technical leader with over 15 years of expertise in distributed systems, data science, machine learning and large-scale data engineering. A patented innovator and published researcher, he specializes in enterprise AI solutions, NLP-driven automation, and Generative AI for business insights. Nirup has led high-impact AI initiatives, including Natural Language to Data systems, enabling businesses to extract actionable insights across multi-source data environments. His expertise spans LLMs, Reinforcement Learning, and Decision Transformers, shaping next-gen AI-driven financial services, retail analytics, and enterprise automation. As a strategic technical leader, he has driven AI adoption at scale, optimizing data architectures, building high-performance ML pipelines, and mentoring cross-functional teams. His work has enhanced real-time decision-making, operational efficiency, and data intelligence for Fortune 500 companies. Nirup brings a wealth of experience across various industries including financial services, retail, and technology.
Comments (0)
This post does not have any comments. Be the first to leave a comment below.
Featured Product
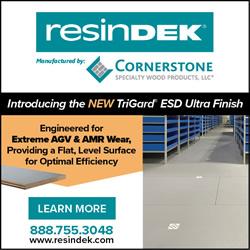